Libraries usage
From HP-SEE Wiki
(→BLAS) |
(→BLAS) |
||
Line 7: | Line 7: | ||
''Section contributed by IPB'' | ''Section contributed by IPB'' | ||
- | + | BLAS (Basic Linear Algebra Subprograms) is a set of routines that provide standard building blocks for performing basic vector and matrix operations. The Level 1 BLAS routines perform scalar, vector and vector-vector operations, the Level 2 BLAS routines perform matrix-vector operations, and the Level 3 BLAS routines perform matrix-matrix operations. BLAS is commonly used in the development software that requires high quality linear algebra routines, as they are efficient, portable, and widely available. The reference BLAS is a freely-available software package and is available for download from the netlib web site ([http://www.netlib.org/blas/ http://www.netlib.org/blas/]). | |
- | Simple BLAS example using the C interface | + | Simple BLAS example using the C BLAS interface ([http://www.netlib.org/blas/blast-forum/cblas.tgz http://www.netlib.org/blas/blast-forum/cblas.tgz]) is illustrated with the code below. It uses <code>DGEMV</code> function to perform a simple matrix-vector multiplication. A corresponding C function from CBLAS is <code>cblas_dgemv</code>. |
#include <stdio.h> | #include <stdio.h> | ||
Line 44: | Line 44: | ||
} | } | ||
- | Machine-specific optimized BLAS libraries are available for a variety of computer architectures. | + | Machine-specific optimized BLAS libraries are available for a variety of computer architectures. Such libraries are provided by the computer vendors or by independent software vendors. Some popular optimized BLAS libraries are: |
* AMD Core Math Library (ACML) - Mathematical library which provides mathematical routines optimized for AMD processors (including a full implementation of Level 1, 2 and 3 Basic Linear Algebra Subroutines). | * AMD Core Math Library (ACML) - Mathematical library which provides mathematical routines optimized for AMD processors (including a full implementation of Level 1, 2 and 3 Basic Linear Algebra Subroutines). | ||
* MKL - Intel's Math Kernel Library of optimized, math routines for science, engineering, and financial applications with math functions which include BLAS, LAPACK, ScaLAPACK, Sparse Solvers, Fast Fourier Transforms, and Vector Math. | * MKL - Intel's Math Kernel Library of optimized, math routines for science, engineering, and financial applications with math functions which include BLAS, LAPACK, ScaLAPACK, Sparse Solvers, Fast Fourier Transforms, and Vector Math. | ||
Line 51: | Line 51: | ||
* Sun Performance Library – A library of optimized subroutines and functions for computational linear algebra and Fourier transforms. It is based on the standard libraries BLAS1, BLAS2, BLAS3, LINPACK, LAPACK, FFTPACK, and VFFTPACK. | * Sun Performance Library – A library of optimized subroutines and functions for computational linear algebra and Fourier transforms. It is based on the standard libraries BLAS1, BLAS2, BLAS3, LINPACK, LAPACK, FFTPACK, and VFFTPACK. | ||
- | Alternatively, user can download ATLAS to automatically generate an optimized BLAS library for | + | Alternatively, a user can download ATLAS to automatically generate an optimized BLAS library for the target architecture. Some pre-built optimized BLAS libraries are also available from the ATLAS site. For a given set of machines, Goto BLAS implementation is also available. |
=== FFTW === | === FFTW === |
Revision as of 18:19, 19 April 2012
Contents |
mpiBLAST
Section to be contributed by SZTAKI
BLAS
Section contributed by IPB
BLAS (Basic Linear Algebra Subprograms) is a set of routines that provide standard building blocks for performing basic vector and matrix operations. The Level 1 BLAS routines perform scalar, vector and vector-vector operations, the Level 2 BLAS routines perform matrix-vector operations, and the Level 3 BLAS routines perform matrix-matrix operations. BLAS is commonly used in the development software that requires high quality linear algebra routines, as they are efficient, portable, and widely available. The reference BLAS is a freely-available software package and is available for download from the netlib web site (http://www.netlib.org/blas/).
Simple BLAS example using the C BLAS interface (http://www.netlib.org/blas/blast-forum/cblas.tgz) is illustrated with the code below. It uses DGEMV
function to perform a simple matrix-vector multiplication. A corresponding C function from CBLAS is cblas_dgemv
.
#include <stdio.h> #include <cblas.h> double m[] = { 3, 1, 3, 1, 5, 9, 2, 6, 5 }; double x[] = { -1, -1, 1 }; double y[] = { 0, 0, 0 }; int main() { int i, j; for (i=0; i<3; ++i) { for (j=0; j<3; ++j) printf("%5.1f", m[i*3+j]); putchar('\n'); } cblas_dgemv(CblasRowMajor, CblasNoTrans, 3, 3, 1.0, m, 3, x, 1, 0.0, y, 1); for (i=0; i<3; ++i) printf("%5.1f\n", y[i]); return 0; }
Machine-specific optimized BLAS libraries are available for a variety of computer architectures. Such libraries are provided by the computer vendors or by independent software vendors. Some popular optimized BLAS libraries are:
- AMD Core Math Library (ACML) - Mathematical library which provides mathematical routines optimized for AMD processors (including a full implementation of Level 1, 2 and 3 Basic Linear Algebra Subroutines).
- MKL - Intel's Math Kernel Library of optimized, math routines for science, engineering, and financial applications with math functions which include BLAS, LAPACK, ScaLAPACK, Sparse Solvers, Fast Fourier Transforms, and Vector Math.
- ESSL - IBM's Engineering and Scientific Subroutine Library, supporting the PowerPC architecture under AIX and Linux.
- libsci - The Cray LibSci library includes a number of parallel numerical libraries including ScaLAPACK, BLAS, BLACS, SuperLU and the Iterative Refinement Toolkit (IRT). The routines in LibSci can be called from both Fortran and C programs.
- Sun Performance Library – A library of optimized subroutines and functions for computational linear algebra and Fourier transforms. It is based on the standard libraries BLAS1, BLAS2, BLAS3, LINPACK, LAPACK, FFTPACK, and VFFTPACK.
Alternatively, a user can download ATLAS to automatically generate an optimized BLAS library for the target architecture. Some pre-built optimized BLAS libraries are also available from the ATLAS site. For a given set of machines, Goto BLAS implementation is also available.
FFTW
Section contributed by IPB
The Fastest Fourier Transform in the West (FFTW) is a comprehensive collection of C routines for computing the discrete Fourier transform (DFT) and its various special cases. Detailed description of FFTW features is available at the official web site (www.fftw.org) and on the HP-SEE wiki Software Stack and Technology Watch / Library (http://hpseewiki.ipb.ac.rs/index.php/Libraries).
Within the NUQG application, FFTW library is used for complex one-dimensional and multi-dimensional DFTs. It is used in serial, OpenMP and MPI parallel mode, as well as in the hybrid OpenMP/MPI mode. Here we describe NUQG FFTW serial and OpenMP usage in 1D, 2D, and 3D, while MPI and hybrid OpenMP/MPI FFTW implementation in NUQG is illustrated in the Hybrid programming and Distributed memory sections, respectively.
The following pseudo-codes show the serial usage of FFTW3 in 1D, 2D, and 3D codes. The codes have to be linked with the fftw3 library -lfftw3 -lm
on Linux/Unix systems. In 1D DFT pseudo-code, Nx is the size of the transform. In multi-dimensional pseudo-codes, Nx and Ny define matrix size in 2D transforms, and Nx, Ny, Nz define tensor size in 3D transforms. FFTW_FORWARD
and FFTW_BACKWARD
tags in plan definitions indicate the direction of the transform. Once the plan has been created, it can be used as many times as needed for transforms on the specified input/output arrays, computing the actual transforms by calling the fftw_execute(plan)
function. The plan is destroyed and the memory used de-allocated by calling the fftw_destroy_plan(plan)
function. Computing the forward transform, followed by the backward transform (or vice versa) yields the original array multiplied by the size of the array Nx (in 1D case), size of the matrix Nx * Ny (in 2D case), or size of the tensor Nx * Ny * Nz (in 3D case), so it is necessary to rescale the data after each transformation.
// FFTW3 serial usage in 1D #include <fftw3.h> int main(int argc, char **argv) { long cnti; long Nx; fftw_complex *array; fftw_plan plan_forward, plan_backward; array = ((fftw_complex *) fftw_malloc(sizeof(fftw_complex) * Nx)); plan_forward = fftw_plan_dft_1d(Nx, array, array, FFTW_FORWARD, FFTW_ESTIMATE); plan_backward = fftw_plan_dft_1d(Nx, array, array, FFTW_BACKWARD, FFTW_ESTIMATE); fftw_execute(plan_forward); fftw_execute(plan_backward); for(cnti = 0; cnti < Nx; cnti ++) { array[cnti][0] /= Nx; array[cnti][1] /= Nx; } fftw_destroy_plan(plan_forward); fftw_destroy_plan(plan_backward); fftw_free(array); }
// FFTW3 serial usage in 2D #include <fftw3.h> int main(int argc, char **argv) { long cnti; long Nx, Ny; fftw_complex *array; fftw_plan plan_forward, plan_backward; array = ((fftw_complex *) fftw_malloc(sizeof(fftw_complex) * Nx * Ny)); plan_forward = fftw_plan_dft_2d(Nx, Ny, array, array, FFTW_FORWARD, FFTW_ESTIMATE); plan_backward = fftw_plan_dft_2d(Nx, Ny, array, array, FFTW_BACKWARD, FFTW_ESTIMATE); fftw_execute(plan_forward); fftw_execute(plan_backward); for(cnti = 0; cnti < Nx * Ny; cnti ++) { array[cnti][0] /= Nx * Ny; array[cnti][1] /= Nx * Ny; } fftw_destroy_plan(plan_forward); fftw_destroy_plan(plan_backward); fftw_free(array); }
// FFTW3 serial usage in 3D #include <fftw3.h> int main(int argc, char **argv) { long cnti; long Nx, Ny, Nz; fftw_complex *array; fftw_plan plan_forward, plan_backward; array = ((fftw_complex *) fftw_malloc(sizeof(fftw_complex) * Nx * Ny * Nz)); plan_forward = fftw_plan_dft_3d(Nx, Ny, Nz, array, array, FFTW_FORWARD, FFTW_ESTIMATE); plan_backward = fftw_plan_dft_3d(Nx, Ny, Nz, array, array, FFTW_BACKWARD, FFTW_ESTIMATE); fftw_execute(plan_forward); fftw_execute(plan_backward); for(cnti = 0; cnti < Nx * Ny * Nz; cnti ++) { array[cnti][0] /= Nx * Ny * Nz; array[cnti][1] /= Nx * Ny * Nz; } fftw_destroy_plan(plan_forward); fftw_destroy_plan(plan_backward); fftw_free(array); }
On the PARADOX cluster, the NUQG application uses FFTW library version 3.3.1. The execution times of the application in 1D, 2D, and 3D as a function of the array size, matrix size, and tensor size are given in the figures below.
The next set of pseudo-codes gives overview of the FFTW OpenMP usage in 1D, 2D, and 3D codes. Applications using the parallel complex transforms should be linked with the switches -lfftw3_threads -lfftw3 -lm
, or -lfftw3_omp -lfftw3 -lm
if the program is compiled with OpenMP. It is also needed to link against the library responsible for threads on your system (e.g. -lpthread
on GNU/Linux), and to include a compiler flag that enables OpenMP (e.g. -fopenmp
with GCC). Before calling any FFTW routines, the fftw_init_threads()
function has to be invoked. This function, which needs to be called only once, performs initialization required for use of threads on the system. It returns zero if an error is encountered, and non-zero value otherwise.
Before creating FFTW3 plan for multi-threaded usage, is is necessary to call the fftw_plan_with_nthreads(int nthreads)
function. The nthreads
argument indicates the number of threads that will be used by FFTW. All plans created subsequently using any of the planner routines will use the same number of threads. Plans already created before a call to the function fftw_plan_with_nthreads
are not affected. If nthreads
is equal to 1 (default), threads are disabled for subsequent plans.
To configure FFTW to use all of the currently running OpenMP threads (set by the omp_set_num_threads(nthreads)
function or by the OMP_NUM_THREADS
environment variable), one should invoke fftw_plan_with_nthreads(omp_get_num_threads())
. The 'omp_
' OpenMP functions are declared via #include <omp.h>
header file.
A given plan can be executed as before, by calling fftw_execute(plan)
, and the execution will use the number of threads specified when the plan was created. The plan can be destroyed the same as in the serial code, by calling fftw_destroy_plan
, while the threads could be de-allocated by calling fftw_cleanup_threads(void)
.
// FFTW3 multi-threaded usage in 1D #include <fftw3.h> int main(int argc, char **argv) { long cnti; long Nx; fftw_complex *array; fftw_plan plan_forward, plan_backward; array = ((fftw_complex *) fftw_malloc(sizeof(fftw_complex) * Nx)); fftw_init_threads(); fftw_plan_with_nthreads(omp_get_max_threads()); plan_forward = fftw_plan_dft_1d(Nx, array, array, FFTW_FORWARD, FFTW_ESTIMATE); plan_backward = fftw_plan_dft_1d(Nx, array, array, FFTW_BACKWARD, FFTW_ESTIMATE); fftw_execute(plan_forward); fftw_execute(plan_backward); for(cnti = 0; cnti < Nx; cnti ++) { array[cnti][0] /= Nx; array[cnti][1] /= Nx; } fftw_destroy_plan(plan_forward); fftw_destroy_plan(plan_backward); fftw_cleanup_threads(); fftw_free(array); }
// FFTW3 multi-threaded usage in 2D #include <fftw3.h> int main(int argc, char **argv) { long cnti; long Nx, Ny; fftw_complex *array; fftw_plan plan_forward, plan_backward; array = ((fftw_complex *) fftw_malloc(sizeof(fftw_complex) * Nx * Ny)); fftw_init_threads(); fftw_plan_with_nthreads(omp_get_max_threads()); plan_forward = fftw_plan_dft_2d(Nx, Ny, array, array, FFTW_FORWARD, FFTW_ESTIMATE); plan_backward = fftw_plan_dft_2d(Nx, Ny, array, array, FFTW_BACKWARD, FFTW_ESTIMATE); fftw_execute(plan_forward); fftw_execute(plan_backward); for(cnti = 0; cnti < Nx * Ny; cnti ++) { array[cnti][0] /= Nx * Ny; array[cnti][1] /= Nx * Ny; } fftw_destroy_plan(plan_forward); fftw_destroy_plan(plan_backward); fftw_cleanup_threads(); fftw_free(array); }
// FFTW3 multi-threaded usage in 3D #include <fftw3.h> int main(int argc, char **argv) { long cnti; long Nx, Ny, Nz; fftw_complex *array; fftw_plan plan_forward, plan_backward; array = ((fftw_complex *) fftw_malloc(sizeof(fftw_complex) * Nx * Ny * Nz)); fftw_init_threads(); fftw_plan_with_nthreads(omp_get_max_threads()); plan_forward = fftw_plan_dft_3d(Nx, Ny, Nz, array, array, FFTW_FORWARD, FFTW_ESTIMATE); plan_backward = fftw_plan_dft_3d(Nx, Ny, Nz, array, array, FFTW_BACKWARD, FFTW_ESTIMATE); fftw_execute(plan_forward); fftw_execute(plan_backward); for(cnti = 0; cnti < Nx * Ny * Nz; cnti ++) { array[cnti][0] /= Nx * Ny * Nz; array[cnti][1] /= Nx * Ny * Nz; } fftw_destroy_plan(plan_forward); fftw_destroy_plan(plan_backward); fftw_cleanup_threads(); fftw_free(array); }
The speedup of FFTW OpenMP code in 1D, 2D, and 3D as a function of the number of CPU cores is shown in the figure below. FFTW 1D code (Nx = 3x10^8) on the PARADOX cluster with 8 CPU cores achieves speedup of 2.1. Better speedup is achieved with FFTW 2D code (Nx = Ny = 2 x 10^4) and FFTW 3D code (Nx = Ny = Nz = 800), 3.5 and 5.8 respectively.
Intel MKL
Section contributed by IPB
Intel Math Kernel Library (Intel MKL) (http://software.intel.com/en-us/articles/intel-mkl/) is a library of optimized, extensively threaded mathematical routines for solving large scientific and engineering computational problems. The library supports C/C++ and Fortran language APIs. The most of Intel MKL functions are threaded, which enables more efficient usage of today’s multi-core processors and easier parallelization of application or implementation of a hybrid programming model. Threading is performed through OpenMP, and the following mathematical domains are supported:
- Sparse Linear Algebra – Sparse BLAS, Sparse Format Converters, PARDISO Direct Sparse Solver, Iterative Sparse Solvers and Pre-conditioners
- Fast Fourier Transforms
- Optimized LINPACK benchmark
- Vector Math Library
- Statistics Functions – Vector Random Number Generators, Summary Statistics Library
- Cluster Support – ScaLAPACK, Cluster FFT
The NUQG application uses Intel MKL Fast Fourier Transform and Trigonometric Transform functionality. The FFTW3 wrappers are a set of functions and data structures, depending on each other. The wrappers are not designed to provide the interface on a function-per-function basis, and some of the wrapper functions are empty and do nothing, but they are present to avoid linking errors and satisfy function calls.
The FFTW3 wrappers provide equivalent implementation for double- and single-precision functions (those with prefixes fftw_
and fftwf_
, respectively). The FFTW3 interface provided by the wrappers is defined in the header files fftw3.h
and fftw3.f
. These files are identical to the ones from the FFTW3.2 package, and are included in the distribution of Intel MKL. Additionally, the files fftw3_mkl.h
, fftw3_mkl.f
, and fftw3_mkl_f77.h
define supporting structures, supplementary constants and macros, and expose Fortran interface in C.
A function call for creation of an FFT plan may return a NULL
plan, indicating that the functionality is not supported. Therefore, it is recommended to carefully check the result returned by a plan creation function within the application. In particular, the following problems return a NULL
plan:
-
c2r
andr2c
problems with a split storage of complex data. -
r2r
problems with kind valuesFFTW_R2HC
,FFTW_HC2R
, andFFTW_DHT
. - Multidimensional
r2r
transforms. - Transforms of multidimensional vectors.
- Multidimensional transforms with rank >
MKL_MAXRANK
.
The new value MKL_RODFT00
is introduced for the kind parameter by the FFTW3 wrappers. For better performance, it is strongly suggested to use this value, rather than the FFTW_RODFT00
:
plan1 = fftw_plan_r2r_1d(n, in1, out1, FFTW_RODFT00, FFTW_ESTIMATE); plan2 = fftw_plan_r2r_1d(n, in2, out2, MKL_RODFT00, FFTW_ESTIMATE);
The flags parameter in plan creation functions is always ignored. The same algorithm is used regardless of the value of this parameter. In particular, flags values FFTW_ESTIMATE
, FFTW_MEASURE
, etc. have no effect.
For multithreaded plans, the normal sequence of calls to the fftw_init_threads()
and fftw_plan_with_nthreads()
functions can be used.
FFTW3 wrappers are not fully thread-safe. If the new-array execute functions, such as fftw_execute_dft()
, share the same plan from parallel user threads, we suggest to set the number of the sharing threads before creation of the plan. For this purpose, the FFTW3 wrappers provide a header file fftw3_mkl.h
, which defines a global structure fftw3_mkl with a field to be set to the number of sharing threads. Below is an example of setting the number of sharing threads:
#include "fftw3.h" #include "fftw3_mkl.h" fftw3_mkl.number_of_user_threads = 4; plan = fftw_plan_dft(...);
The wrappers typically indicate a problem by returning a NULL
plan. In few cases, the wrappers may report a descriptive message of the problem detected. By default, the reporting is turned off. To turn it on, one needs to set a variable fftw3_mkl.verbose
to the non-zero value, for example:
#include "fftw3.h" #include "fftw3_mkl.h" fftw3_mkl.verbose = 0; plan = fftw_plan_r2r(...);
Intel MKL Fast Fourier Transform and Trigonometric Transform functionality is used by NUQG and tested on the PARADOX cluster. First, we have compared execution times of serial codes that use FFTW3 3.1.2 library compiled with the GCC compiler, compiled with the ICC compiler, as well as FFTW library provided by Intel MKL 10.2.3. The execution times are shown in the figure below as a function of a number of performed forward and backward DFT transforms. The size of the data array is 10^7. As we can see, there is no significant improvement in performance when the FFTW3 3.1.2 library is compiled with ICC instead of GCC. However, some improvement in performance is achieved when Intel MKL FFTW library is used.
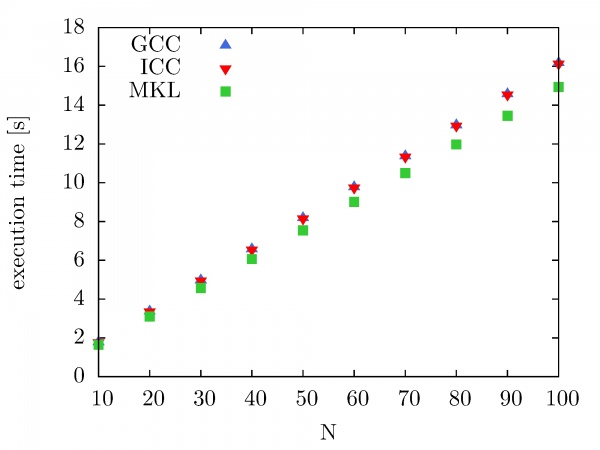
The next figure illustrates the speedup of the Intel MKL Fast Fourier Transform library on the PARADOX cluster as a function of the number of CPU cores used. In this case, the same data array size is used (10^7), while the number of performed forward and backward DFT transforms has been varied from 100 to 1000, with a step of 100. As we can see, the speedup of Intel MKL FFTW library on the PARADOX cluster is 3.8 for 4 CPU cores, and 5.8 for 8 CPU cores.
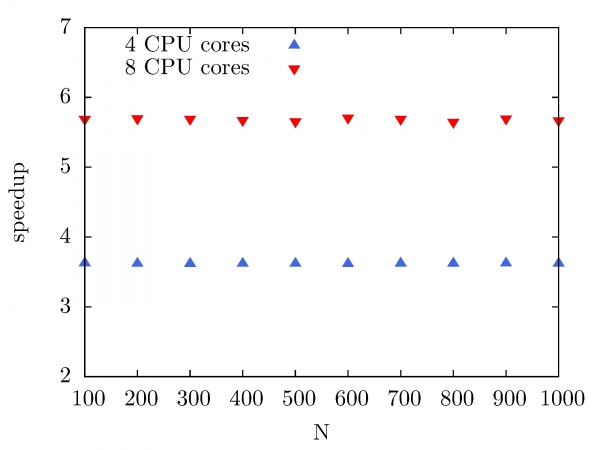
SPRNG
Section contributed by IPB
Pseudorandom number generation algorithms are key components in many modern scientific computing applications and the quality of the obtained research results often critically depends on their characteristics. The random number generating algorithms should be of sufficiently high quality, so as to ensure that numerical results give unbiased and reliable estimates of physical quantities. SPRNG (Scalable Parallel Random Number Generators) is one of the best libraries for generating pseudorandom numbers for parallel applications. Detail description of SPRNG features is available at the official web page (sprng.fsu.edu) and at the HP-SEE Wiki Software Stack and Technology Watch / Library (http://hpseewiki.ipb.ac.rs/index.php/Libraries) section.
Two versions of the SPRNG library are available on the PARADOX cluster: SPRNG 2.0 and SPRNG 4.0. The NUQG application uses SPRNG 4.0 library, both in the serial and in the MPI mode for the Monte Carlo calculation of path integrals of a generic quantum mechanical theory. Typical usage in the serial mode is illustrated with the following pseudo-code:
#include <sprng_cpp.h> #define SEED 12345 int main() { int streamnum, nstreams; double rn; int irn; Sprng * stream; streamnum = 0; nstreams = 1; stream = SelectType(SPRNG_CMRG); stream->init_sprng(streamnum, nstreams, SEED, SPRNG_DEFAULT); // Generate a double precision random number rn = stream->sprng(); // Generate an integer random number irn = stream->isprng(); // Free memory used to store stream state ptr->free_sprng(); }
The SPRNG MPI usage is illustrated with the following pseudo-code:
#include <sprng_cpp.h> #define SEED 12345 int main(int argc, char *argv[]) { int streamnum, nstreams; Sprng *stream; double rn; int myid, nprocs; // Initialize MPI MPI_Init(&argc, &argv); MPI_Comm_rank(MPI_COMM_WORLD, &myid); MPI_Comm_size(MPI_COMM_WORLD, &nprocs); streamnum = myid; // One stream per processor nstreams = nprocs; //node 0 is reading in a generator type stream = SelectType(SPRNG_CMRG); // Initialize stream stream->init_sprng(streamnum,nstreams,SEED,SPRNG_DEFAULT); // Generate double precision random number rn = stream->sprng(); // Free memory used to store stream state stream->free_sprng(); // Terminate MPI MPI_Finalize(); }
The NUQG Monte Carlo (MC) algorithm uses SPRNG streams independently, with almost no interprocess communication, and therefore the scaling of MPI NUQG MC algorithm with the number of processors is perfectly linear.
CPMD
Section contributed by IPB
The CPMD code is a parallelized plane-wave/pseudopotential implementation of the Density Functional Theory (DFT), particularly designed for ab-initio molecular dynamics. CPMD runs on a large variety of different computer architectures and is optimized to run on either (super-)scalar or vector CPUs. General description of CPMD and the list of features are available at the official web site (cpmd.org) and at the HP-SEE Wiki Software Stack and Technology Watch / Library section http://hpseewiki.ipb.ac.rs/index.php/CPMD.
CPMD is ported on the PARADOX cluster with MPI parallelization. The application is fully functional and can be started with the following command:
[dusan@ui ~]$ cpmd.x file.in [PP_path] > file.out
where file.in
is an input file and [PP_path]
is the location of pseudopotential files for all atomic species specified in the file.in
input file.
The path to the pseudopotential library can be given in different ways:
- By setting the second command line argument
[PP_path]
. - If the second command line argument
[PP_path]
is not given, the program checks the environment variablesCPMD_PP_LIBRARY_PATH
andPP_LIBRARY_PATH
. - If neither the environment variables nor the second command line argument are set, the program assumes that the pseudopotential files reside in the current directory.
During the run, cpmd.x
creates different outputs:
- Various status messages to monitor the correct operation of the program is printed to standard output (in our case, redirected to the file
file.out
).
- Detailed data are written into different files (depending on the keywords specified in the input
file.in
). Large files are written either to the current directory, the directory specified by the environment variableCPMD_FILEPATH
, or the directory specified in the input file using the keywordFILEPATH
.
The CPMD official web site provides several CPMD introduction guides:
- Wavefunction Optimization Example - demonstrate some of the basic steps of performing a CPMD calculation with a very simple molecule: hydrogen, and a very simple task: calculate the electronic structure.
http://cpmd.org/cpmd-tutorial/the-basics-running-cpmd-input-and-output-formats
- Geometry Optimization and MD Example - inspect how to perform a geometry optimization and how to setup and analyze a Car-Parrinello MD.
http://cpmd.org/cpmd-tutorial/the-basics-of-cpmd-geometry-optimization-and-md
- Electron Structure and Geometry Optimization Example - gives three exercises (Hydrogen molecule, Water and Ammonia) to practice with the basics of CPMD.
http://cpmd.org/cpmd-tutorial/exercise-1-electron-structure-and-geometry-optimization
- Car-Parrinello MD Example - explain how to setup a Car-Parrinello Molecular Dynamics run.
http://cpmd.org/cpmd-tutorial/exercise-2-car-parrinello-md
- Bulk Systems Example - look into more complex systems like bulk materials.
http://cpmd.org/cpmd-tutorial/exercise-3-bulk-systems
- Determination of Dynamical Properties Example - is a collection of some examples on how to determine and tune dynamical properties in CPMD.
http://cpmd.org/cpmd-tutorial/exercise-4-determination-of-dynamical-properties
- Proton transfer in an Enzyme Example - shows how to setup/run a model system for a real-life simulation.
http://cpmd.org/cpmd-tutorial/exercise-5-triade
GAMESS
Section contributed by IICT-BAS
The GAMESS application was used by the MD Cisplatin application. In order to explore the capabilities of the free quantum-chemical software Firefly PCGamess, appropriate purely organic molecules were chosen, with the aid, on a later stage the program to be applied to metal-containing systems, like cisplatin analogues. The work done has thus an important methodological significance. Molecular mechanics conformational search for the two species was performed, and the global minimum-energy conformations thus obtained, were further optimized at HF ab initio (3-21G** basis set, Firefly PCGamess) level of theory. In both cases, specific, scorpion-like conformations are realized, with hydrogen bonds involving the guanidino-group and the phenolic hydroxyl. The application was porting to MPI using GAMESS application software on the HPCG cluster at IICT-BAS. A lot of MPI tests were done up to 32 cores to optimize the application. A good parallel efficiency is obtained up to 32 cores on HPCG cluster. Some problems were solved by testing different versions of Gamess software application and selecting the version that achieves correct execution. This may be due to some over-optimisation by the compiler. The GAMESS application is rather flexible and during configuration phase one can select different standard libraries and compiler flags. All tasks using Gamess were compiled on the HPCG cluster using Intel Math Kernel Library, which resulted in good overall performance.
libtiff
Section contributed by UVT
FuzzyCmeans used a special library, libtiff, to deal with the digital images involved in the computational process. Libtiff (http://www.libtiff.org/) was compiled for BG/P@UVT and InfraGRID cluster without any specific optimizations.
The workflow dealing with satellite images (most of the time in TIFF format) using libtiff library is the following:
1. open the TIFF image in memory
TIFF* image; if((image=TIFFOpen(name , "w")) == NULL) { printf("Error open the output file"); return -1; }
2. read image header and set some global variables for later use (image coordinates, image size, image bands etc.)
TIFFGetField(image, TIFFTAG_BITSPERSAMPLE, BPS); TIFFGetField(image, TIFFTAG_SAMPLESPERPIXEL, SPP); TIFFGetField(image, TIFFTAG_ROWSPERSTRIP, RPS); TIFFGetField(image, TIFFTAG_XRESOLUTION, XR); TIFFGetField(image, TIFFTAG_YRESOLUTION, YR); TIFFGetField(image, TIFFTAG_RESOLUTIONUNIT, RuN); TIFFGetField(image,TIFFTAG_IMAGEWIDTH, width); TIFFGetField(image,TIFFTAG_IMAGELENGTH, height);
3. read image values (define a structure that can hold the entire image, read each pixel value and assign it to the tiff structure)
uint32 *raster= (uint32*) _TIFFmalloc(width*height*sizeof(uint32)); // read the entire image if(TIFFReadRGBAImageOriented(image, width, height, raster, ORIENTATION_TOPLEFT, 1)) { // process the raster to compute the image }
4. when all computations are done then write the virtual image from memory on the disk and free up memory
char *buffer; TIFF* image; buffer=malloc(width*height*sizeof(char)); // open a new file on disk for writting if((image=TIFFOpen(name , "w")) == NULL) { printf("Error open the output file"); return -1; } // set initial TIFF headers TIFFSetField(image,TIFFTAG_IMAGEWIDTH, width); TIFFSetField(image,TIFFTAG_IMAGELENGTH, height); TIFFSetField(image, TIFFTAG_BITSPERSAMPLE, 8); TIFFSetField(image, TIFFTAG_SAMPLESPERPIXEL, 1); TIFFSetField(image, TIFFTAG_ROWSPERSTRIP, TIFFDefaultStripSize(image, 0)); TIFFSetField(image, TIFFTAG_PHOTOMETRIC, PHOTOMETRIC_MINISWHITE); TIFFSetField(image, TIFFTAG_FILLORDER, FILLORDER_MSB2LSB); TIFFSetField(image, TIFFTAG_PLANARCONFIG, PLANARCONFIG_CONTIG); TIFFSetField(image, TIFFTAG_XRESOLUTION, XR); TIFFSetField(image, TIFFTAG_YRESOLUTION, YR); TIFFSetField(image, TIFFTAG_RESOLUTIONUNIT, RuN); // For each pixel find the closest center and set the corresponding gray level in the output image for(i=0;i<height*width;i++) { // fill the buffer with gray level values … Buffer[i] = value; … } // write values to the file for(i=0;i<height;i++) { TIFFWriteScanline(image,&buffer[i*width], i,0); } TIFFClose(image);
The snippets presented are only parts of the entire program and must be used as an usage example. For more details please consult the library API.